By: Cheng Lai Ki

The digital-physical divide is shrinking, evident from the increased incorporation of cloud computing alongside an exponential increase in development of smart systems and products. With billions of people connected to the internet today, this globalized informatization can be represented as an ‘Internet-of-Things’ (IoT). IoT can be defined as the nexus of smart devices connected to the internet through inbuilt components, supporting or augmenting end-user experiences and communication. Within the last decade alone, cyberspace has revolutionized our recreational experiences, corporate decision-making and national security services. In a period dominated by data and hyper-connectivity, consumers, developers and service providers all share the responsibility to understand the main cybersecurity trends of today. Here, we address three major cybersecurity trends of IoT-Data Security, Non-Viral Threats, and Evolving Computerized Solutions.
IoT-Data Security
In August 2016, Prof. Yuval Noah Harari from the Hebrew University of Jerusalem suggested the emergence of a new market called Data-ism. All human activity– especially in an IoT-driven landscape – can be deconstructed into quantifiable elements and is subsequently collated by Big Data companies. There is a reason why Google services (i.e. Gmail, Chrome, and GoogleEarth) are all free. Every piece of commercial or recreational software and smart product in our possession generates data, which is crunched through analytics software and sent back to the developers so they may streamline their marketing strategies. This was also visible in the political domain when big-league prognosticators failed to take into account for ‘uncertainties of their [predictive] models’, which lead to a miscalculation about the outcome of the 2016 Presidential Election in the United States. Everything you do is collated as data and subsequently delivered to marketers for targeted advertising purposes – which is actually all stated in their notoriously long Terms of Service agreements.[1]
Mentioned earlier, a characteristic of our IoT environment is cloud computing and the marketization of software, information and platforms as services by large tech companies such as IBM or Google. The expedited convenience towards information sharing and processing has made the cloud an increasing attraction to Data Analytic firms. The attractiveness of convenience has stimulated a growing trend, ‘with 74% [stating that] they expect to adopt a hybrid or cloud-only approach to analytics over the next three years’.[2] While the incorporation of cloud computing remains attractive to large organizations, the technical infrastructure required has become commoditized as a service – which presents its own vulnerabilities.
Earlier this year, there has been an increased cyber-attacks targeting user data. This places everyone’s information at significant risk to being extracted by cyber criminals and distributed for profit, especially to service providers with a heavy reliance on cloud computing. The broad application characteristics enabled in our IoT landscape enabled through cloud computing have since become an increasing focus of cybersecurity companies in providing secure services and Information Security (InfoSec) solutions.
Non-Viral Threats & Ransomware
Within the continuous emergence of new recreational and threat-detection software and platforms, cybercriminals are evolving their tactics when conducting Computer Network Exploitations (CNEs). Over the last decade, cybercriminals have begun to incorporate script-based exploits, where commands are injected directly into the firmware of targeted Operating Systems (OS). The broad application windows programs have led malicious state and non-state actors to conduct CNEs using PowerShell, JavaScript or VBScript. Through using command and control elements already present, attackers are able to quickly access and hijack targeted OS. As the exploit is not a virulent code, older or obsolete anti-virus programs could not detect such CNE tactics – until recently.
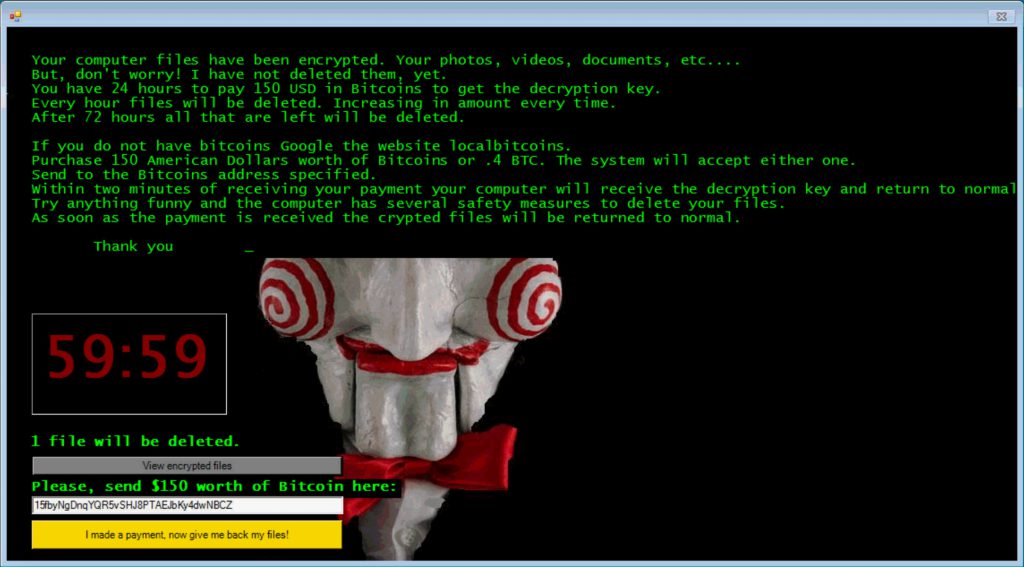
Such covert CNEs would mean attackers could easily gain access to their targeted computer networks and initiate their main objectives. According to a 2016 Symantec ISTR Special Report, crypto-ransomware has emerged as ‘one of the most dangerous cyber threats facing both organizations and consumers’.[3] Crypto-ransomware is when the attacker hijacks and encrypts the files or OS and – as the name suggests – holds it for a monetary fee. While these originated as crimes of opportunity, Symantec discovered that business organizations are increasingly targeted through sophisticated CNE tactics rivaling those utilized in state-level cyberespionage. In addition, their incident response teams also discovered that crypto-ransomware have also been used as a Distributed Denial of Service (DDoS) distraction to cover up other CNEs.
Ergo, the next cybersecurity trend is the increased focus on developing tools (i.e. Threat Detection and Monitoring Interfaces) to identify script-based CNEs alongside other advanced persistent threats (APTs) involving crypto-ransomware or other DDoS techniques.
Evolving Computerized Solutions
It was predicted in 2012 that Internet traffic would reach 1.3 Zettabytes by 2016.[4] Adding onto that, this series has revealed how interconnected our IoT-driven world is. As the size of our networks grow, so does the need for consistent and omnipotent security. Currently, cybersecurity firms are continuously developing and perfecting programs for threat detection or monitoring and data management such as Splunk. The latest ‘trend’ within these domains is the emergence of advanced computerized cybersecurity countermeasures powered through Machine Learning and Deep Learning.
Machine Learning (ML) represents the development of computerized programs that could learn and evolve from its exposure to new data. ML solutions are developed with specialized ‘algorithms [designed] to build a model from example inputs to [develop] data-driven predictions or decisions’.[5] Cybersecurity companies such as Cylance are investing a significant amount of research into applying ML towards advancing cybersecurity analytics and encapsulating upon the magnitude and value of our data-driven world.
Teetering closer to artificial intelligence is Deep Learning (DL), an advanced computerization reflecting the neural networks of the human brain and mimicking its ‘ability to learn and identify objects’.[6] DL solutions are more advanced and capable to self-developing new recognition algorithms, resulting in higher processing functions. Most cyber threats are modified versions of other malware or older variants.
Recorded information of older malware gives DL solutions a checklist of search parameters which mimics how human cybersecurity experts can recognize recycled code or scripts. Currently, cybersecurity firm Deep Instinct is the first company to pioneer DL into a cybersecurity platform.

Unlike ML, DL requires minimal human input and possesses a significantly higher degree of self-sustainability, thereby reducing the propensity of human error when developing recognition algorithms to identify APTs and other CNE characteristics. Regardless, either solution provides cybersecurity technicians and incident response teams expedited abilities to address intrusions quickly. This makes such solutions highly valuable to commercial and governmental security agencies coordinating large amounts of (perhaps sensitive or classified) data and requiring extensive InfoSec services.
Singularity & Security
Throughout this series, we have fundamentally addressed the power of software, the emerging vulnerabilities of robotic platforms, the vulnerabilities of limited information and platform security, and the importance of cyber crisis-management. Within the current cybersecurity landscape today, it can be identified that the industry is focusing on cultivating STEM (Science, Technology, Engineering and Mathematics) personnel capable of handling the technical domains within cybersecurity – due to an apparent lack of skilled personnel to match increasingly sophisticated threats. Given the increasing sophistication of cyber criminals and APTs, the demand for technical specialists is understandable.[7] However, we also need to cultivate personnel who are capable of understanding and deploying cybersecurity measures from a strategic and policy level.
Cybersecurity is in itself a reflection of singularity, the integration of man and machine. Where technical specialists, ML and DL solutions can provide critical information to deployment specialists to develop and implement human-element strategies for national and commercial infrastructures. Cyberspace is not only ubiquitous but has become an integral element of the modern world, supporting national security, military intelligence, business exchanges, global data management and critical infrastructures. In practice, the threats are consistently evolving, requiring specialist knowledge in multiple domains. This series has shown that all a malicious actor essentially needs is one vulnerability to initiate a CNE. Hence, we all share the responsibility to welcome a secure digital future.
Cheng is a graduate from the MA Intelligence and International Security program at King’s College London, his Master’s thesis examined the characteristics and trends defining China’s emerging cybersecurity and cyberwarfare capabilities. He was a finalist at the 2016 Cyber 9/12 Student Challenge in Geneva, contributed to other security journals such as IHSJane’s Intelligence Review and was a Former Managing Editor (Blog) at Strife.
Notes:
[1] Lee, W. & Rotoloni, B. Emerging Cyber Threats Report 2016, (Georgia Tech Cyber Security Summit 2015: Georgia Institute of Technology), (2015); [Online]; Available from: http://www.iisp.gatech.edu/sites/default/files/documents/2016_georgiatech_cyberthreatsreport_onlinescroll.pdf, (Accessed 1 Nov 2016).
[2] An ISTR Special Report: Ransomware and Businesses 2016, Symantec, (2016).
[3] 1 Zettabyte = 1,000,000,000,000,000,000,000 Bytes
[4] Newman, S. ‘Intro to Machine Learning & Cybersecurity: 5 Key Steps’, DARKReading, (7 September 2015); [Online]; Available from: http://www.darkreading.com/analytics/intro-to-machine-learning-and-cybersecurity-5-key-steps-/a/d-id/1322539, (Accessed 1 November 2016).
[5] Caspi, G. ‘Introducing Deep Learning: Boosting Cybersecurity Within an Artificial Brain’, DARKReading, (9 June 2016); [Online]; Available from: http://www.darkreading.com/analytics/introducing-deep-learning-boosting-cybersecurity-with-an-artificial-brain/a/d-id/1326824, (Accessed 13 October 2016).
[6] Goodman, M. Future Crimes: Inside the Digital Underground and the battle for our Connected World, (Transworld Publishers: London, UK), 2015.
[7] ‘15% of the 200 IT [Information Technology] and business decision markers in the survey say they have deployed one or more cloud analytics solutions…[and] with 68% of respondents saying they intend to investigate, analyze or actively plan to deploy analytics solutions over the coming year.
Image 1 credit: http://map.norsecorp.com/#/
Image 2 credit: http://blog.trendmicro.com/trendlabs-security-intelligence/jigsaw-ransomware-plays-games-victims/
Image 3 credit: https://www.datanami.com/2015/11/16/machine-learnings-big-role-in-the-future-of-cybersecurity/, (Accessed 1 November 2016)

Cheng Lai Ki
Cheng Lai Ki is a Freelance Intelligence Analyst in Singapore and works in the field of cybersecurity, geopolitical risk and international security. He has an MA in Intelligence and International Security from King's College London and was a former Managing Editor at StrifeBlog.